Introduction: The Elusive Quest for Contextual Understanding in NLP
Imagine a world where your virtual assistant doesn’t just hear your words—it truly understands the context behind them, down to the images you’re looking at or the tone of your voice. In 2025, Natural Language Processing (NLP) has transformed how we interact with technology, powering everything from chatbots to translation apps. Yet, despite these leaps, a fundamental challenge persists: context. A phrase like “I’m feeling blue” could mean sadness or literally wearing blue, depending on the situation. Traditional NLP models, which rely solely on text, often miss these nuances, leading to awkward misunderstandings that remind us how far AI still has to go. But what if there’s a game-changer on the horizon? Multimodal NLP, which integrates text, images, and potentially other data types, promises to crack this puzzle by 2030. Could this be the breakthrough that brings AI closer to human-like understanding, or are we chasing an impossible dream?
What Is Multimodal NLP, and Why Does It Matter?
Multimodal NLP isn’t just a buzzword—it’s a revolution in how machines interpret the world. Unlike traditional NLP, which processes language in isolation, multimodal NLP combines multiple data streams to create a richer picture of meaning. Picture this: you’re texting a friend about a recent trip, saying, “This view was incredible!” while sharing a photo of a sunset over the ocean. A traditional NLP model might interpret your message generically, missing the visual context. A multimodal model, however, can analyze the image alongside your text, understanding that “this view” refers to a specific scene—a fiery orange sky melting into the horizon. By 2025, models like Google’s MUM and Meta AI’s VisualBERT are already blending text and images to power smarter search engines and conversational agents. But here’s the provocative question: if machines can “see” and “read” together, are we on the verge of AI that truly gets us—or are we just teaching them to mimic our perception without understanding it?
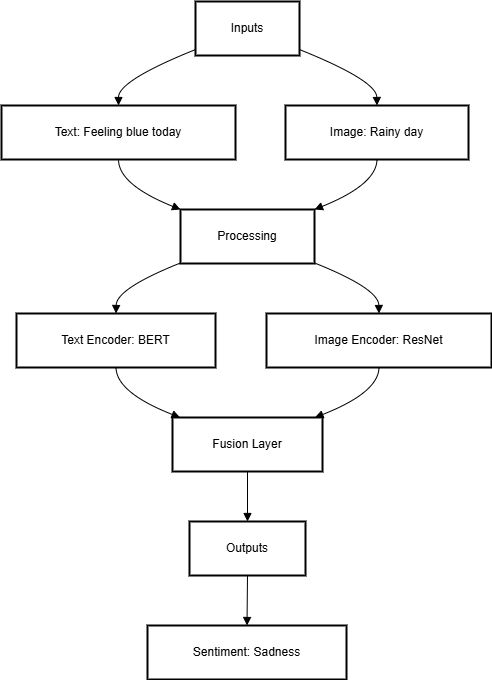
The Promise of Multimodal NLP: A Glimpse into the Future
Let’s fast-forward to 2030. Imagine a classroom where a student asks, “What’s the difference between these two plants?” while pointing to a diagram in their textbook. A multimodal NLP-powered chatbot doesn’t just hear the question—it sees the diagram, identifies the plants, and delivers a detailed comparison in seconds. In healthcare, doctors could describe symptoms while uploading scans, and AI systems could cross-reference both to suggest diagnoses with pinpoint accuracy. In entertainment, filmmakers might use multimodal AI to generate scripts that perfectly match visual storyboards, blending creativity with precision. These scenarios aren’t far-fetched—they’re the future multimodal NLP could unlock. Models in 2025 are already using transformer architectures to align text and visual embeddings, creating a unified understanding of meaning. But here’s the thought-provoking twist: if AI can understand context this deeply, are we creating machines that rival human intuition, or are we just crafting better illusions of understanding?
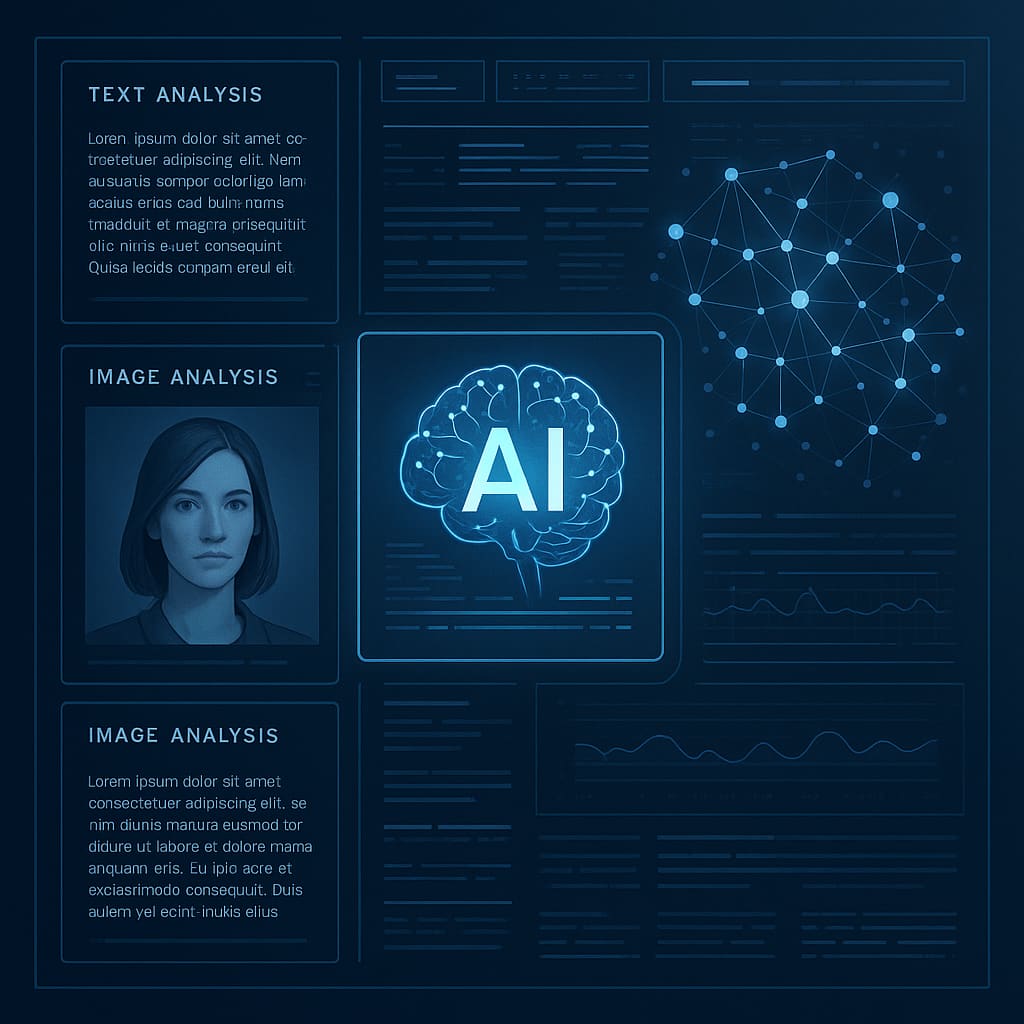
The Challenges: Can We Overcome the Hurdles by 2030?
The path to 2030 is fraught with challenges that could derail this vision. First, multimodal NLP models are resource-intensive, requiring immense computational power to train and deploy. In 2025, training a model like MUM can cost millions of dollars and consume energy equivalent to a small city’s daily usage—a sustainability nightmare. Second, aligning text and images isn’t as simple as it sounds. A model might misinterpret an image due to poor lighting, cultural differences in visual symbols, or even deliberate manipulation, leading to flawed conclusions. Third, there’s the ethical minefield: if training data contains biases (and most do), multimodal models could amplify these biases, misinterpreting contexts in ways that reinforce stereotypes or spread misinformation. For example, a model trained on biased image-text pairs might associate certain professions with specific genders, skewing its understanding of context. Can we address these hurdles—computational, technical, and ethical—in just five years, or will they stall multimodal NLP’s progress?
Potential Impact on AI Applications: A Double-Edged Sword
If multimodal NLP fulfills its promise by 2030, the impact on AI applications could be seismic. In education, multimodal chatbots could revolutionize learning, interpreting both written questions and visual aids to provide personalized, context-aware responses. In healthcare, AI systems could analyze patient reports alongside medical imaging, offering diagnoses that rival human doctors. In entertainment, multimodal AI could generate films where scripts, visuals, and soundtracks are seamlessly aligned, redefining creativity. But there’s a darker side to consider: as AI understands context better, it also gains the ability to manipulate it. Imagine a multimodal model crafting hyper-realistic fake news, combining text and images to deceive millions. Or consider privacy—what happens when AI can infer deeply personal contexts from your photos and conversations? The same technology that empowers us could also exploit us, raising profound questions about the role of AI in society.
Conclusion: A Future of Possibility and Peril
Multimodal NLP stands at the crossroads of possibility and peril. By 2030, it could solve the contextual understanding problem, bringing AI closer to human-like intuition and transforming industries from education to entertainment. Yet, the challenges—computational costs, alignment issues, and ethical risks—loom large. As we race toward this future, we must ask ourselves: are we building machines that truly understand us, or are we creating tools that can mimic understanding while amplifying our flaws? The answers will shape not just the future of NLP, but the future of AI itself, challenging us to balance innovation with responsibility in a world where context is everything.
Comments