Introduction: The Big Data Boom and Its Hidden Cost
In 2025, machine learning (ML) has become the backbone of innovation, driving everything from personalized ads to self-driving cars. The fuel behind this revolution? Big Data. With global data creation projected to exceed 180 zettabytes this year, ML models have access to more information than ever before, enabling breakthroughs that were once unimaginable. Models like Google’s BERT and OpenAI’s GPT series owe their success to massive datasets, learning patterns that make them seem almost human. But here’s the unsettling question: are we paying a hidden price for this data deluge? As datasets grow larger, the risk of overfitting looms larger too, threatening to undermine the very advancements we celebrate. Are we building ML models that truly understand the world, or are we just teaching them to memorize the noise in our data?
Understanding Overfitting: When Models Learn Too Much
Overfitting is the dark shadow of machine learning success. It happens when a model learns the training data too well, capturing not just the underlying patterns but also the noise, outliers, and quirks specific to that dataset. The result? A model that performs flawlessly on the training set but fails miserably on new, unseen data. Imagine training an ML model on billions of social media posts to predict user behavior. It might nail the training data, predicting likes and shares with eerie precision—until it encounters a new user whose behavior doesn’t match the dataset’s trends. Suddenly, the model flounders, unable to generalize. In 2025, the stakes are higher than ever, as Big Data amplifies this risk. With datasets so vast and complex, models can easily latch onto meaningless patterns, like a child memorizing answers without understanding the lesson. Is Big Data leading us to smarter AI, or just better memorizers?
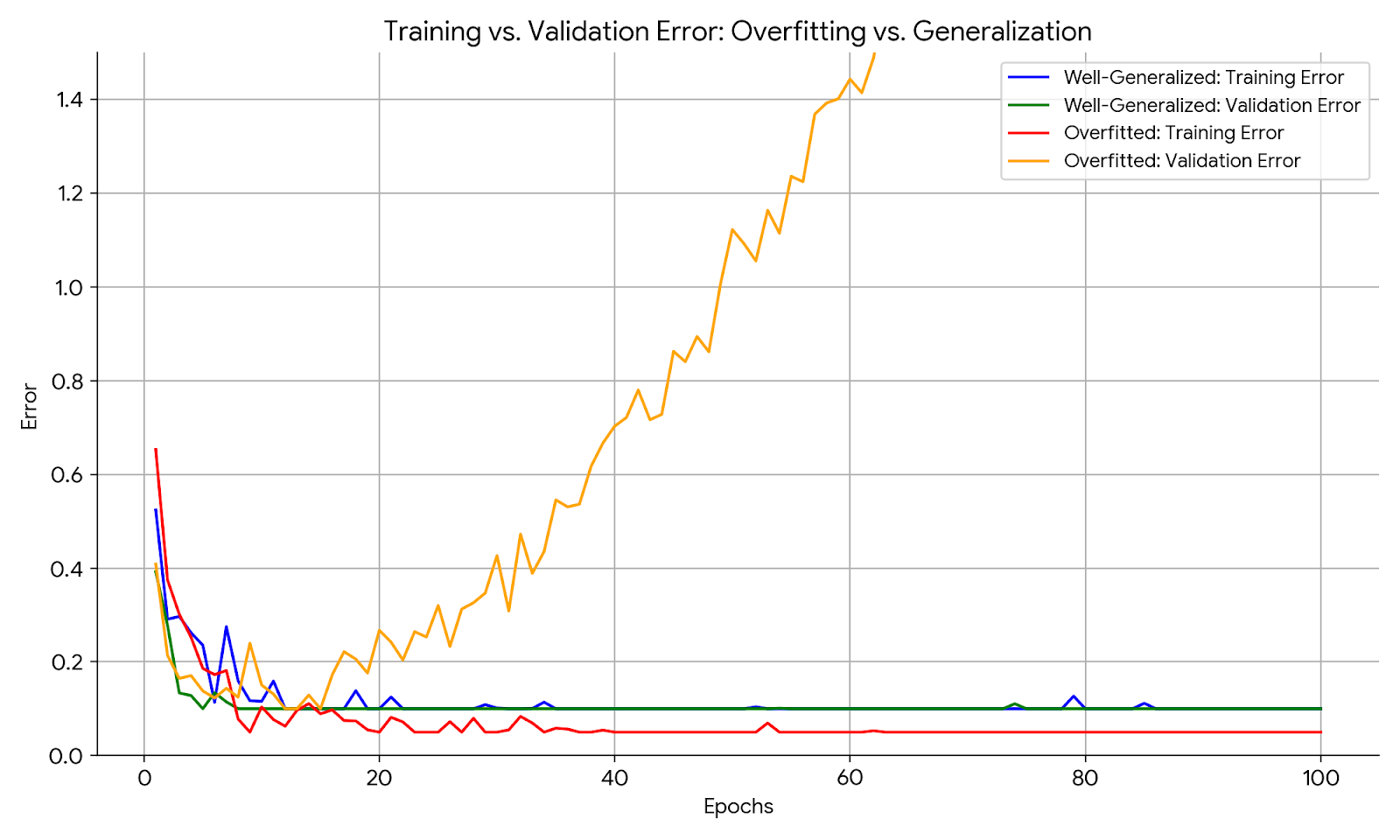
The Challenges: Why Big Data Makes Overfitting Worse
In 2025, Big Data is both a blessing and a curse. On one hand, it provides the raw material for ML models to learn intricate patterns. On the other, it creates new challenges that make overfitting more likely. First, the sheer scale of data overwhelms traditional regularization techniques like dropout or weight decay. Large datasets often contain redundant or noisy data—think billions of internet photos with varying quality, lighting, and angles. An image recognition model might overfit to specific camera artifacts or lighting conditions, failing to recognize the same object in a different setting. Second, the computational cost of training on Big Data is staggering, making it harder to experiment with multiple models or hyperparameters to curb overfitting. Third, ethical concerns loom large: if a dataset reflects societal biases (e.g., overrepresentation of certain demographics), an overfitted model might perpetuate those biases, leading to unfair predictions in critical applications like hiring or lending. Are we sacrificing fairness and reliability for the allure of more data?
Solutions: Can We Tame the Beast of Big Data?
The ML community in 2025 isn’t sitting idle—innovative solutions are emerging to tackle overfitting in the age of Big Data. One promising approach is data pruning: before training even begins, algorithms can filter out irrelevant or noisy data points, ensuring models focus on high-quality, informative samples. Active learning takes this a step further, prioritizing data that challenges the model to learn better generalizations. Another solution lies in advanced regularization techniques, like stochastic depth (randomly dropping layers during training) or mixup (blending images and labels to create synthetic data), which introduce randomness to prevent models from overfitting. Federated learning offers a decentralized approach, training models across distributed datasets without centralizing data, reducing the risk of overfitting to a single, biased source. Finally, tools like SHAP (SHapley Additive exPlanations) help us peek inside models, revealing when they’re relying too heavily on specific features—a sign of overfitting. But here’s the provocative question: can these solutions keep pace with the exponential growth of data, or are we fighting a losing battle against complexity?
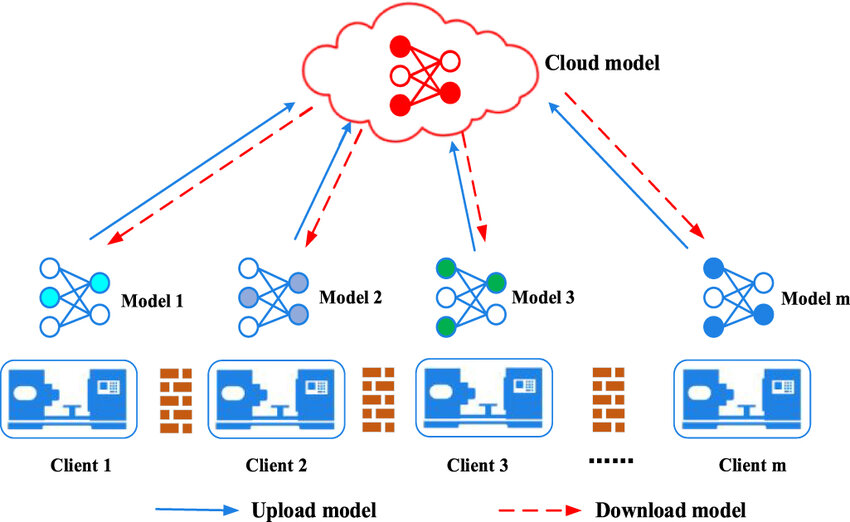
The Future: A Balancing Act for Machine Learning
As we look to the future of machine learning in 2025 and beyond, the challenge of overfitting to Big Data will define the field’s trajectory. Researchers are exploring data-efficient learning techniques like few-shot learning, where models learn from just a handful of examples, mimicking how humans generalize from limited experience. Synthetic data generation—using generative AI to create diverse, high-quality datasets—could also reduce reliance on massive, noisy data, offering a controlled way to train models. But here’s the deeper issue: are we focusing too much on data quantity over quality? Perhaps the future of ML lies not in bigger datasets, but in smarter ones—curated, diverse, and designed to teach models how to think, not just memorize. If we can strike this balance, ML in 2025 could deliver AI that’s not only powerful but also trustworthy, capable of navigating the real world with human-like adaptability.
Conclusion: A Call to Rethink Big Data’s Role in ML
Big Data has propelled machine learning to new heights, but it’s a double-edged sword. In 2025, the risk of overfitting threatens to undermine these gains, creating models that excel in the lab but falter in the wild. Solutions like data pruning, advanced regularization, and federated learning offer hope, but they also force us to confront a bigger question: are we using Big Data as a crutch, hoping sheer volume will solve problems that require deeper ingenuity? The future of ML depends on our ability to rethink how we harness data—not just collecting more, but using it wiser. As we navigate this challenge, we might find that the path to truly intelligent AI lies not in bigger data, but in better thinking.
Comments