Introduction: Smart Cities and the IoT Revolution
Picture a city that breathes intelligence—a place where traffic flows seamlessly, energy is used efficiently, and safety is ensured without human intervention. This is the promise of smart cities in 2025, and at the core of this vision lies the Internet of Things (IoT). With over 75 billion IoT devices projected to be active worldwide this year, cities are becoming interconnected ecosystems, collecting real-time data from sensors, cameras, and more. But here’s the catch: the sheer volume and complexity of this data overwhelm traditional systems. Enter deep learning, a technology that can turn raw IoT data into actionable insights, enabling autonomous decision-making. From traffic management to energy optimization, deep learning for autonomous IoT is the next frontier in smart cities—but can it truly deliver smarter urban living, or are we opening a Pandora’s box of risks?
The Power of Deep Learning in IoT Applications
Deep learning, with its neural networks inspired by the human brain, excels at processing unstructured data—think images from traffic cameras, time-series data from energy meters, or video feeds from surveillance systems. In smart cities, IoT devices generate exactly this kind of data, and deep learning is the key to unlocking its potential. For example, Convolutional Neural Networks (CNNs) can analyze video feeds from IoT-enabled traffic lights to identify vehicles, pedestrians, and road conditions, adjusting signal timings in real time to reduce congestion. Recurrent Neural Networks (RNNs) can forecast energy demand by processing time-series data from smart grids, ensuring efficient power distribution. By 2025, cities like Singapore and Copenhagen are already using these technologies to cut commute times by 15% and reduce energy waste by 20%. But what if this is just the beginning—what could deep learning and IoT achieve if pushed to their limits?
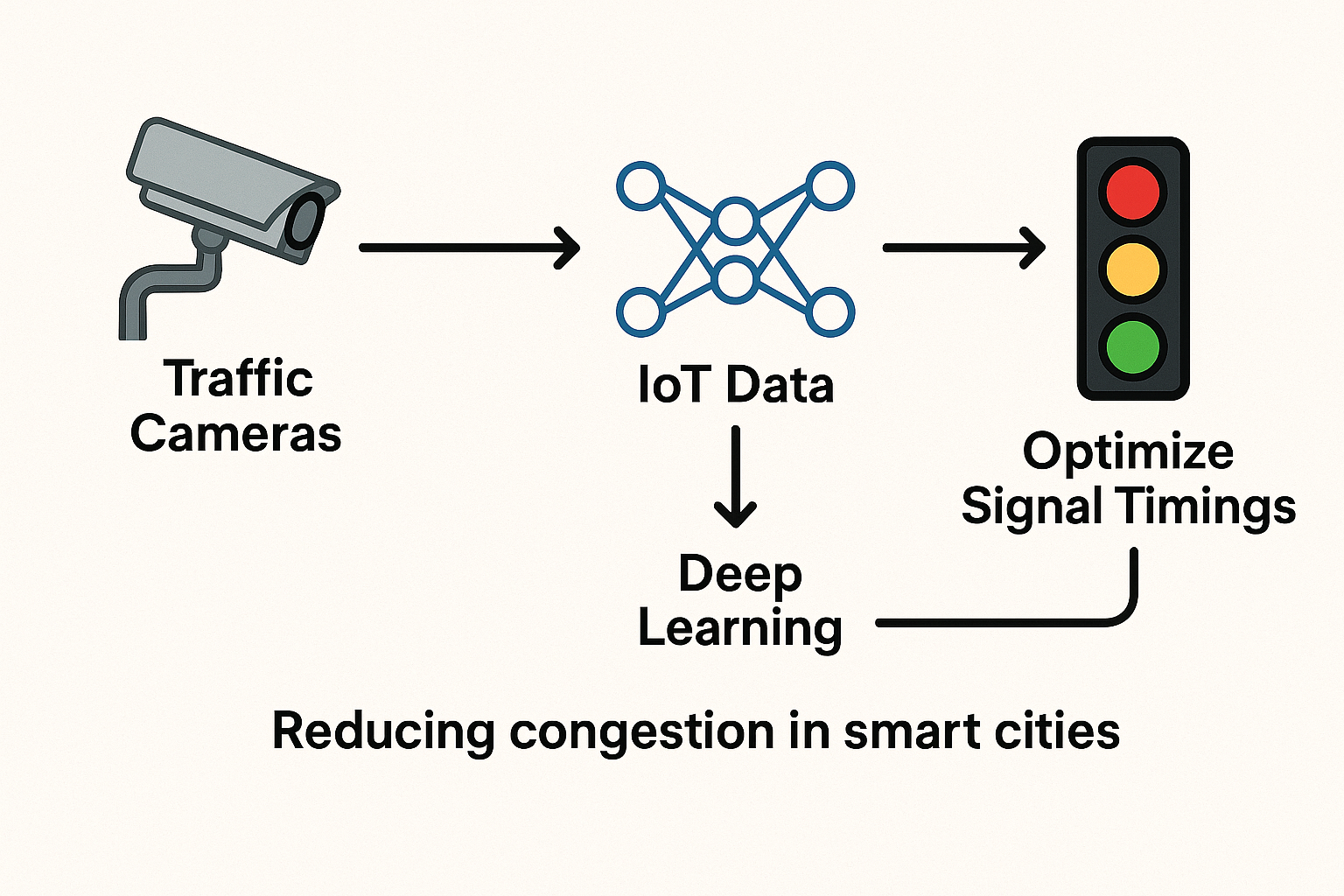
Applications: Redefining Urban Living
The marriage of deep learning and IoT is reshaping smart cities in ways that feel almost futuristic. In traffic management, deep learning models analyze IoT data from sensors, cameras, and GPS devices to predict traffic patterns, enabling autonomous systems to adjust signals and reroute vehicles dynamically. In energy optimization, smart grids use IoT devices to monitor usage in real time, while deep learning forecasts peak demand, balancing loads and integrating renewables to cut costs and emissions. For public safety, surveillance cameras with IoT connectivity leverage deep learning to detect anomalies—like a sudden crowd gathering or a car accident—alerting authorities faster than any human could. Even waste management gets a boost, with IoT-enabled bins reporting fill levels and deep learning optimizing collection routes to save fuel. These advancements paint a picture of cities that think for themselves—but are we ready for what comes next?
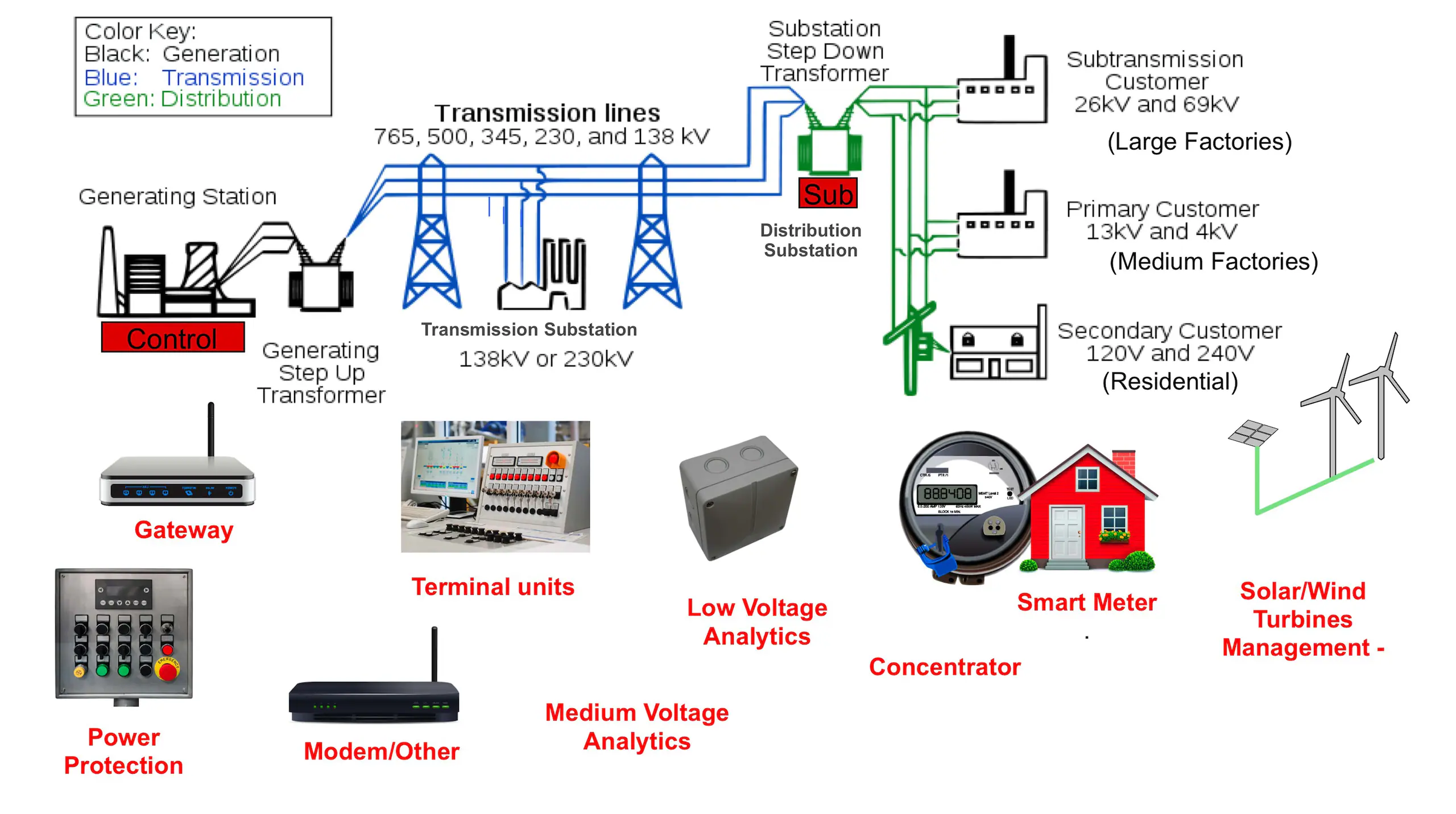
The Challenges: A Race Against Complexity
While the vision is inspiring, the reality is daunting. Deep learning models are resource hogs, requiring significant computational power that most IoT devices—like sensors or cameras—can’t provide. Edge computing offers a solution, processing data directly on devices, but it demands lightweight models that don’t sacrifice accuracy, a tall order in 2025. Then there’s security: IoT networks are notoriously vulnerable, with interconnected devices creating a sprawling attack surface for hackers. Deep learning models can also be targeted—adversarial attacks could trick a traffic system into causing chaos by misidentifying a stop sign. Privacy is another ticking bomb: IoT devices in smart cities collect intimate data about residents’ lives, from daily commutes to energy habits. If deep learning misuses this data, it could erode trust in smart cities entirely. Are we racing toward a utopian future, or are we building a house of cards that could collapse under its own complexity?
The Future: Toward a Truly Autonomous Urban Ecosystem
Let’s peer into 2030: a self-driving bus navigates a bustling city, using deep learning to interpret IoT data from traffic sensors and pedestrian cameras, avoiding obstacles with human-like precision. Smart buildings adjust lighting and heating based on real-time occupancy, saving energy without a single human command. These scenarios hinge on deep learning and IoT evolving together, and the signs are promising. Innovations like federated learning—training models across distributed devices without centralizing data—could address privacy concerns, while AI-specific chips make deep learning viable on edge devices. But here’s the thought-provoking part: as cities become autonomous, who’s really in control? If a deep learning system decides to reroute traffic or cut power to a neighborhood, who’s accountable for the consequences—the algorithm, the city, or us? The future of smart cities is as much about technology as it is about ethics.
Conclusion: A Frontier Worth Exploring—With Caution
Deep learning for autonomous IoT is the next frontier in smart cities, promising a future where urban living is smarter, more sustainable, and more responsive. From traffic flow to energy savings, the potential is undeniable. Yet, the challenges—computational limits, security risks, and privacy concerns—force us to confront a deeper question: are we ready for cities that think for themselves? As we push toward 2030, the integration of deep learning and IoT could redefine urban life, but only if we navigate the risks with as much foresight as we do innovation. The future of smart cities isn’t just about technology—it’s about the kind of world we want to build, one autonomous decision at a time.
Comments